A review on statistical noise models for Magnetic Resonance Imaging
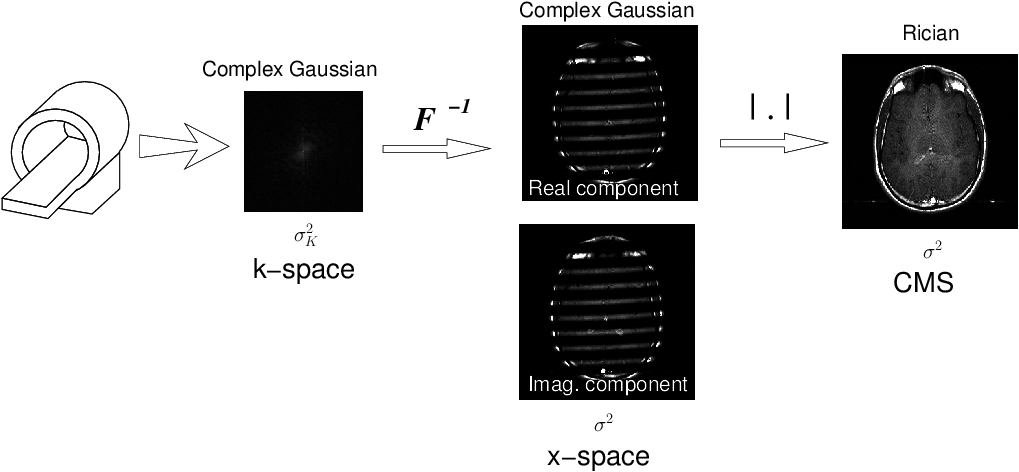
TECH-LPI2013-01 (V2.0). This work is an original Technical Report of the LPI, Universidad de Valladolid, Spain, Jun. 2013 (Update Nov. 2015).
Abstract: Many processing applications within MRI are grounded on stochastic methods based on the prior knowledge on the statistics of noise. In order to properly tune any method to the specific data, it is therefore necessary to identify the specific noise model present. The probability model of noise in the MRI data will depend on the coil configuration of the scanner and on the kind of processing the MR data goes through before producing the final magnitude image. The noise in MRI has been commonly modeled as a stationary process governed by a Rician distribution with constant noise power at each voxel. However, modern MRI systems turn this model questionable, making it necessary to develop into more complex patterns. The manipulation of the original Gaussian data, the combination of different coil information and adaptive processing change the features of noise changing the underlying statistical models as well as the stationarity assumption. This work aims at comprehensively reviewing the main statistical rationales and formulations for the noise in MRI lately found in the literature, attending to the first-order voxel-wise probability law and the possible spatial variability of the parameters of such distribution. For all the statistical models we assume that: (1) data are acquired using a regular Cartesian sampling; (2) the different contributions of noise are all independent; and (3) post processing schemes are not applied. The implications of the reviewed models over real applications are discussed. Finally, we explore the applicability of the surveyed models to some MRI protocols commonly used.
Key words: MRI, thermal noise, noise modeling, Rician, non-central Chi, parallel MRI.